- From www.coursera.org
Probabilistic Graphical Models 2: Inference
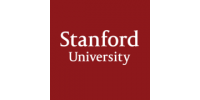
- Self-paced
- Free Access
- Fee-based Certificate
- 5 Sequences
- Advanced Level
Course details
Syllabus
- Week 1 - Inference Overview
This module provides a high-level overview of the main types of inference tasks typically encountered in graphical models: conditional probability queries, and finding the most likely assignment (MAP inference). - Week 1 - Variable Elimination
This module presents the simplest algorithm for exact inference in graphical models: variable elimination. We describe the algorithm, and analyze its complexity in terms of properties of the graph structure. - Week 2 - Belief Propagation Algorithms
This module describes an alternative view of exact inference in graphical models: that of message passing between clusters each of which encodes a factor over a subset of variables. This framework provides a basis for a variety of exact and approximate inferen... - Week 3 - MAP Algorithms
This module describes algorithms for finding the most likely assignment for a distribution encoded as a PGM (a task known as MAP inference). We describe message passing algorithms, which are very similar to the algorithms for computing conditional probabilitie... - Week 4 - Sampling Methods
In this module, we discuss a class of algorithms that uses random sampling to provide approximate answers to conditional probability queries. Most commonly used among these is the class of Markov Chain Monte Carlo (MCMC) algorithms, which includes the simple G... - Week 4 - Inference in Temporal Models
In this brief lesson, we discuss some of the complexities of applying some of the exact or approximate inference algorithms that we learned earlier in this course to dynamic Bayesian networks. - Week 5 - Inference Summary
This module summarizes some of the topics that we covered in this course and discusses tradeoffs between different algorithms. It also includes the course final exam.
Prerequisite
Instructors
Daphne Koller
Professor
School of Engineering
Editor
La Leland Stanford Junior University, plus connue sous le nom d'université Stanford, est une université américaine privée, située dans la Silicon Valley au sud de San Francisco.
Sa devise est « Die Luft der Freiheit weht » qui signifie « Le vent de la liberté souffle ».
Arrivant parmi les premières universités au monde dans la plupart des classements internationaux, elle jouit d'un grand prestige.
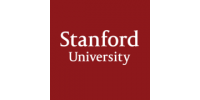
Platform
Coursera - это цифровая компания, предлагающая массовые открытые онлайн-курсы, основанные учителями компьютеров Эндрю Нгом и Стэнфордским университетом Дафни Коллер, расположенные в Маунтин-Вью, штат Калифорния.
Coursera работает с ведущими университетами и организациями, чтобы сделать некоторые из своих курсов доступными в Интернете, и предлагает курсы по многим предметам, включая: физику, инженерию, гуманитарные науки, медицину, биологию, социальные науки, математику, бизнес, информатику, цифровой маркетинг, науку о данных и другие предметы.
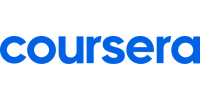