- 来自www.coursera.org
Algorithmic Thinking (Part 1)
课程
en
英语
字幕可用
40 时
此内容评级为 0/5
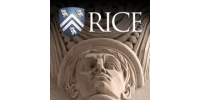
- 自定进度
- 免费获取
- 收费证书
- 4 序列
- 等级 中级
- 字幕在 Greek, Spanish
课程详情
教学大纲
- Week 1 - Module 1 - Core Materials
What is Algorithmic Thinking?, class structure, graphs, brute-force algorithms - Week 2 - Modules 1 - Project and Application
Graph representations, plotting, analysis of citation graphs - Week 3 - Module 2 - Core Materials
Asymptotic analysis, "big O" notation, pseudocode, breadth-first search - Week 4 - Module 2 - Project and Application
Connected components, graph resilience, and analysis of computer networks
先决条件
没有。
讲师
Luay Nakhleh
Associate Professor
Computer Science; Biochemistry and Cell Biology
Scott Rixner
Professor
Computer Science
Joe Warren
Professor
Computer Science
编辑
莱斯大学是一所美国私立研究型大学,位于德克萨斯州休斯敦市。它位于该市的博物馆区,毗邻德克萨斯医学中心。莱斯大学由威廉-马什-莱斯(William Marsh Rice,en)于 1891 年创建,当时名为 "威廉-马什-莱斯文学、科学和艺术发展学院"(The William Marsh Rice Institute for the Advancement of Letters, Science, and Art),1912 年落成,埃德加-奥德尔-洛维特(Edgar Odell Lovett)担任首任校长。
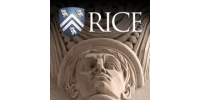
平台
Coursera是一家数字公司,提供由位于加利福尼亚州山景城的计算机教师Andrew Ng和达芙妮科勒斯坦福大学创建的大型开放式在线课程。
Coursera与顶尖大学和组织合作,在线提供一些课程,并提供许多科目的课程,包括:物理,工程,人文,医学,生物学,社会科学,数学,商业,计算机科学,数字营销,数据科学 和其他科目。
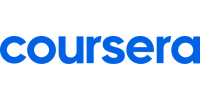
完成这个资源,写一篇评论