- From www.udacity.com
High Performance Computing
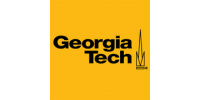
- Self-paced
- Free Access
- 24 Sequences
- Introductive Level
Course details
Syllabus
Prerequisite
Instructors
- Rich Vuduc - Rich Vuduc an associate professor in the School of Computational Science and Engineering (CSE) atGeorgia Tech. His research is in the area of high-performance computing.This year, Professor Vuduc is also serving as both the Associate Chair of Academic Affairs in the School of CSE and as the Director of CSE Programs.Research: The HPC Garage [@hpcgarage].Professor Vuduc’s lab is developing automated tools and techniques to tune, to analyze, and to debug software for parallel machines, including emerging high-end multi/manycore architectures and accelerators. They focus on applying these methods to CSE applications, which include computer-based simulation of natural and engineered systems and data analysis.
Editor
The Georgia Institute of Technology, also known as Georgia Tech or GT, is a co-educational public research university located in Atlanta, Georgia, USA. It is part of the wider University System of Georgia network. Georgia Tech has offices in Savannah (Georgia, USA), Metz (France), Athlone (Ireland), Shanghai (China), and Singapore.
Georgia Tech's reputation is built on its engineering and computer science programmes, which are among the best in the world5,6. The range of courses on offer is complemented by programmes in the sciences, architecture, humanities and management.
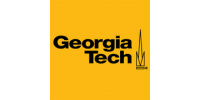
Platform
Udacity is a for-profit educational organization founded by Sebastian Thrun, David Stavens, and Mike Sokolsky offering massive open online courses (MOOCs). According to Thrun, the origin of the name Udacity comes from the company's desire to be "audacious for you, the student". While it originally focused on offering university-style courses, it now focuses more on vocational courses for professionals.
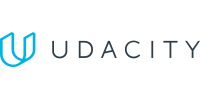