- From www.edx.org
Successfully Evaluating Predictive Modelling
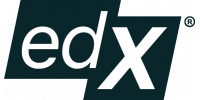
- 6 Sequences
- Advanced Level
- Starts on October 25, 2021
- Ends on December 19, 2021
Course details
Syllabus
Week 1: Evaluation Metrics and Feature Selection
Week 2: Feature Selection and Correlation Analysis
Week 3: Feature Selection with Decomposition Techniques
Week 4: Sampling Techniques
Week 5: Resampling Techniques
Week 6: Case Study
Prerequisite
You should be familiar with an undergraduate level, or have a background, in mathematics and statistics. Previous experience with a procedural programming language is beneficial (e.g. Python, C, Java, Visual Basic).
Learners pursuing the MicroMasters programme are strongly recommended to complete PA1.1x Introduction to Predictive Analytics using Python on the verified track prior to undertaking this course.
Instructors
Dr Xuefei Lu
Lecturer in Predictive Analytics
University of Edinburgh
Sofia Varypati
Course Tutor
University of Edinburgh
Obinna Unigwe
Course Tutor
The University of Edinburgh
Dr Johannes De Smedt
Assistant Professor in Business Information Systems
KU Leuven
Dr Zexun Chen
Lecturer in Predictive Analytics
University of Edinburgh
Platform
Harvard University, the Massachusetts Institute of Technology, and the University of California, Berkeley, are just some of the schools that you have at your fingertips with EdX. Through massive open online courses (MOOCs) from the world's best universities, you can develop your knowledge in literature, math, history, food and nutrition, and more. These online classes are taught by highly-regarded experts in the field. If you take a class on computer science through Harvard, you may be taught by David J. Malan, a senior lecturer on computer science at Harvard University for the School of Engineering and Applied Sciences. But there's not just one professor - you have access to the entire teaching staff, allowing you to receive feedback on assignments straight from the experts. Pursue a Verified Certificate to document your achievements and use your coursework for job and school applications, promotions, and more. EdX also works with top universities to conduct research, allowing them to learn more about learning. Using their findings, edX is able to provide students with the best and most effective courses, constantly enhancing the student experience.
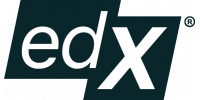